All
Assume Nothing
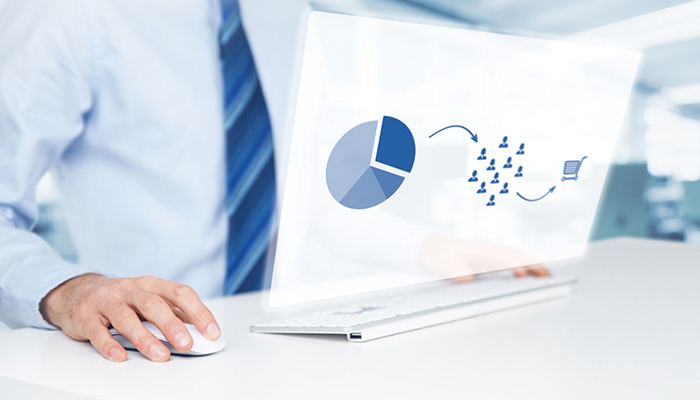
Predictive analytics depend on customer data, not marketer presumptions
There are two things energy marketers know about their new customers, according to Phil Baratz, president of Angus Energy. First, they know that they don’t know much about new customers. Second, they know that they definitely don’t know how new customers will behave in the weeks, months and (hopefully) years to come.
Frustrating as it may be, this Socratic paradox actually provides a window of opportunity for the open-minded energy marketer, as it encourages one to disregard assumptions and embrace the power of cold, hard data. This was the central theme of “Unlock Your Customer’s Secrets. Your data already knows,” a presentation given by Baratz at the 2017 Eastern Energy Expo and NEFI EXPO.
Referencing a model that included some 18,000 retail energy customers, Baratz discussed the increasing value and precision of predictive analytics for the home comfort provider. Using third-party software, Angus Analytics was able to model some 36 duodecillion customer data combinations in 28 seconds, Baratz explained. (One duodecillion equals 1039.) “That’s far better than gut instinct and far better than previous models that used only eight categories of customers,” he said.
In the past, Baratz reasoned, the prevailing thought was that if a company had enough “good” and “bad” new customers — respectively, those who will stay for 5-15 years and those who won’t — the company would do OK for itself. Today, he said, it’s more important for a company to identify the “good” customers as soon as possible, so that the company can allocate its resources toward keeping them, thus defending its market share.
In order to determine which new customers are “good” and which are “bad,” predictive analytics must utilize “all data” rather than fill in the gaps with “assumed data,” Baratz said. This is done in order to avoid predictive bias. “If you’re looking for something, you’ll find that thing,” Baratz explained. Data miners should, however, disregard useless data such as social security numbers and generic dates (like January 1, 1900).
Once Angus Analytics had all of its “clean data” in place, it was able to build its model. What that model revealed may come as a big surprise for fuel dealers still operating under common industry assumptions. Here are just a few of its findings:
- Higher credit scores are not great indicators of customer loyalty. Customers with decent credit (not perfect and not terrible) are most likely to stay.
- A lack of service complaints does not indicate customer satisfaction or loyalty. The notion that a customer will be happier if the company never has to service their equipment didn’t hold up.
- The most predictive factors of customer loyalty were service contract enrollment, years as a customer and settlement price. The longer customers stay, the better the chance they’ll stay even longer.
Related Posts
8 Strategies for Effective Wealth Transfer in a Business Succession
Posted on June 16, 2025
What Do Drivers and Technicians Want?
Posted on May 14, 2025
Long-Term Demand and Equipment Investments
Posted on May 13, 2025
Show Me the Money
Posted on April 28, 2025
Enter your email to receive important news and article updates.